Unlocking Business Potential with Machine Learning Labeling Tools
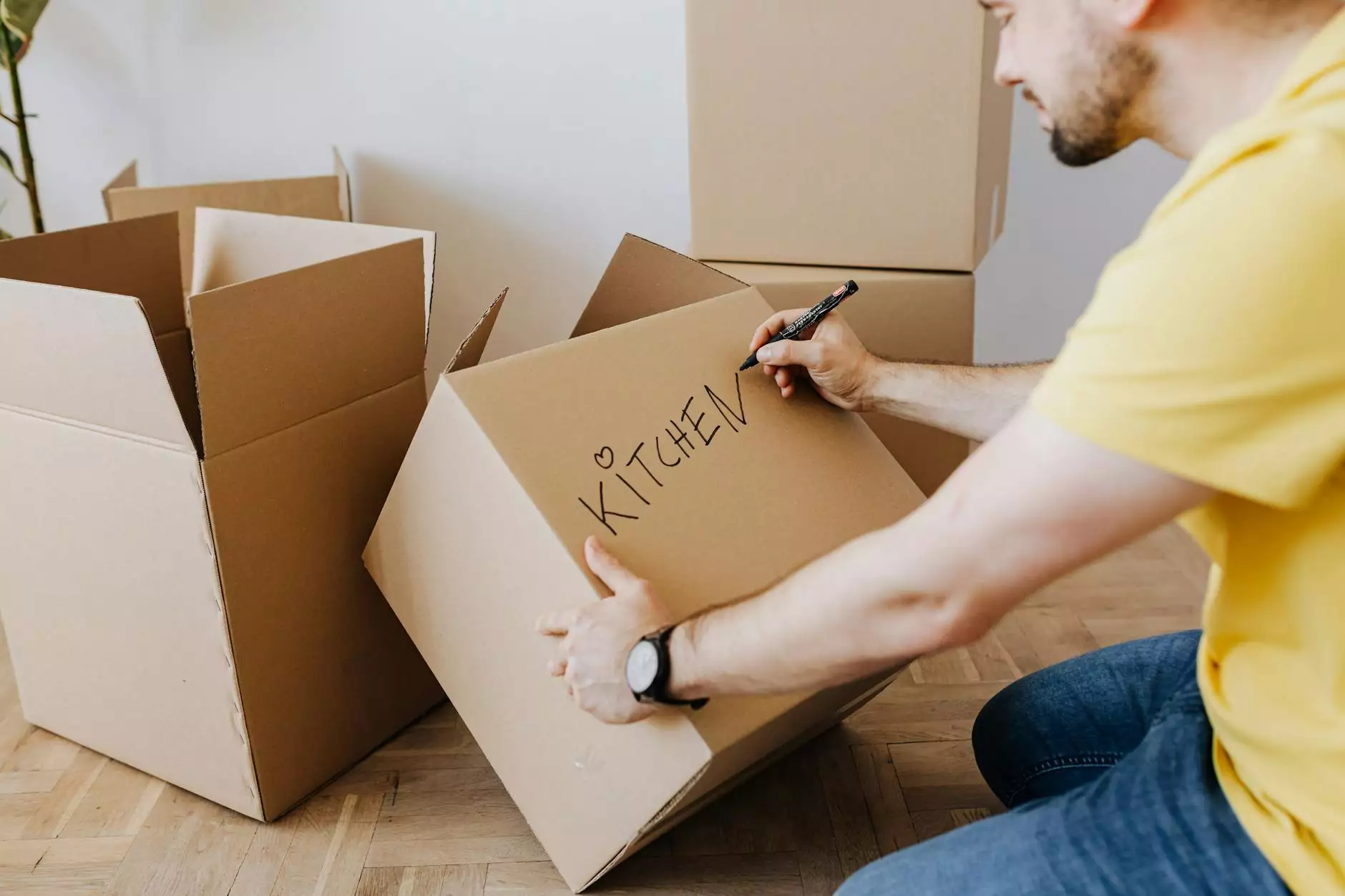
In the digital age, data is more than just a collection of numbers and facts; it's a vital asset that can drive innovation and fuel growth. Businesses are increasingly turning to machine learning labeling tools as a means to streamline their data annotation processes. These tools not only enhance the efficiency of data management but also improve the accuracy of machine learning models, thus paving the way for significant advancements in various industries.
The Importance of Data Annotation
Data annotation is the process of labeling data to make it understandable for machine learning models. It's a crucial step in developing algorithms that can learn from data. Without accurately annotated data, the machine learning models can produce skewed results that can lead to poor decision-making. Here are some key points highlighting the importance of data annotation:
- Improves Model Accuracy: Annotated data allows machine learning algorithms to learn from correct examples, significantly improving the accuracy of predictions.
- Facilitates Effective Training: High-quality labeled data ensures that the model can generalize well to new, unseen data.
- Enables Advanced Insights: Clear data categorization helps businesses derive meaningful insights that can inform strategies and decision-making processes.
Understanding Machine Learning Labeling Tools
Machine learning labeling tools encompass various software and platforms designed to facilitate the data annotation process. These tools automate the tedious and often manual task of labeling, allowing businesses to focus on higher-level strategy and deployment of machine learning solutions. Here are some features that make these tools invaluable:
Key Features of Machine Learning Labeling Tools
- User-Friendly Interface: Most high-end labeling tools provide an intuitive interface that simplifies the annotation process, making it accessible to non-technical users.
- Automation Capabilities: These tools often come with machine learning-assisted features that can predict labels, significantly speeding up the annotation process.
- Customization: Businesses can customize their labeling tasks, accommodating different types of data such as images, text, and videos.
- Collaboration Features: Multiple users can work on the same project, allowing teams to collaborate in real-time and improve overall productivity.
- Quality Control: Many tools offer built-in quality control checks to ensure that the annotations are accurate and reliable.
Types of Data Annotation Tools
When considering data annotation tools, it’s essential to understand the different types available and their respective applications:
1. Image Annotation Tools
These tools are specifically designed for labeling images. They can be used in various applications such as autonomous vehicles, healthcare, and retail. Common techniques include:
- Bounding Boxes: Drawing rectangles around objects to identify them.
- Segmentation: Pixel-level labeling to divide an image into different segments.
2. Video Annotation Tools
Video annotation is critical for training models that rely on motion detection. These tools allow for the tagging of objects throughout video frames, which is especially useful in areas like surveillance and sports analytics.
3. Text Annotation Tools
Text annotation is vital for natural language processing (NLP) applications. Tools may provide functionalities for:
- Entity Recognition: Identifying and labeling specific entities within text, such as names, dates, and organizations.
- Sentiment Analysis: Labeling text with sentiment indicators (positive, negative, neutral).
Benefits of Using Machine Learning Labeling Tools
Integrating machine learning labeling tools into your business operations can lead to a myriad of benefits. Here’s how they can transform your data practices:
1. Enhanced Efficiency
By automating the annotation process, these tools significantly reduce the time and labor costs associated with manual labeling. This efficiency enables teams to handle larger datasets and complete projects faster.
2. Improved Data Quality
Quality is paramount when it comes to data annotations. Machine learning labeling tools often incorporate error-checking algorithms and collaborative review processes to ensure high-quality output.
3. Scalability
As your business grows, so does your data. Leveraging a sophisticated labeling tool allows for easy scalability. You can handle increased volumes of data without compromising on quality or efficiency.
4. Cost-Effectiveness
Investing in a machine learning labeling tool can reduce costs related to hiring a large workforce for manual annotations. Automating the process leads to significant savings in time and resources.
5. Better Focus on Core Business Activities
With the heavy lifting of data annotation handled by a tool, your team can redirect their focus toward core business strategies, improving overall productivity and innovation.
Choosing the Right Machine Learning Labeling Tool
Selecting the right machine learning labeling tool requires careful consideration of your business needs and goals. Here are key factors to consider:
1. Compatibility
Ensure that the tool you choose is compatible with your existing systems and can effectively handle the types of data you work with.
2. User Support
Good customer support is essential. Look for tools that provide comprehensive documentation, tutorials, and responsive customer support teams.
3. Pricing Structure
Evaluate the pricing model of the tool. Some tools charge based on the volume of data or number of users, while others may have a flat rate.
4. Security Features
Data security is critical. Choose a solution that offers robust security features to protect your sensitive information during the annotation process.
Case Studies: Success with Machine Learning Labeling Tools
To understand the real-world impact of machine learning labeling tools, let’s explore some case studies where businesses have successfully utilized these platforms:
Case Study 1: Healthcare Sector
A healthcare provider used an image annotation tool to train its AI model for detecting tumors in MRI scans. By accurately labeling a dataset of thousands of scans, the model achieved over 90% accuracy in predictions, significantly improving patient outcomes.
Case Study 2: Retail Industry
A major retail brand implemented a text annotation tool to analyze customer feedback. By labeling sentiments and key themes in customer reviews, the brand gained insights that shaped their marketing strategies and product developments, leading to increased customer satisfaction.
Future Trends in Machine Learning Labeling Tools
The landscape of machine learning labeling tools is evolving rapidly. Here are some future trends to watch out for:
- Integration of AI: The next generation of labeling tools will likely rely more on AI to predict and automate labeling tasks further.
- Focus on Collaborative Annotation: As remote work becomes the norm, tools that facilitate collaborative annotation will become increasingly essential.
- Enhanced Visualization: As data complexity grows, enhanced data visualization features will help annotators better understand what they are labeling.
Conclusion
Investing in a machine learning labeling tool is not merely a trend; it's a strategic move that can significantly elevate your business's data handling capabilities. As we’ve explored, the right tool can enhance data quality, improve efficiency, and potentially lower costs. In today’s data-driven world, embracing these tools will empower businesses to harness the full potential of their data, leading to smarter decisions and greater success.
If you are looking for an exceptional data annotation tool or data annotation platform to enhance your operations, consider visiting KeyLabs.ai for innovative solutions tailored to your needs.